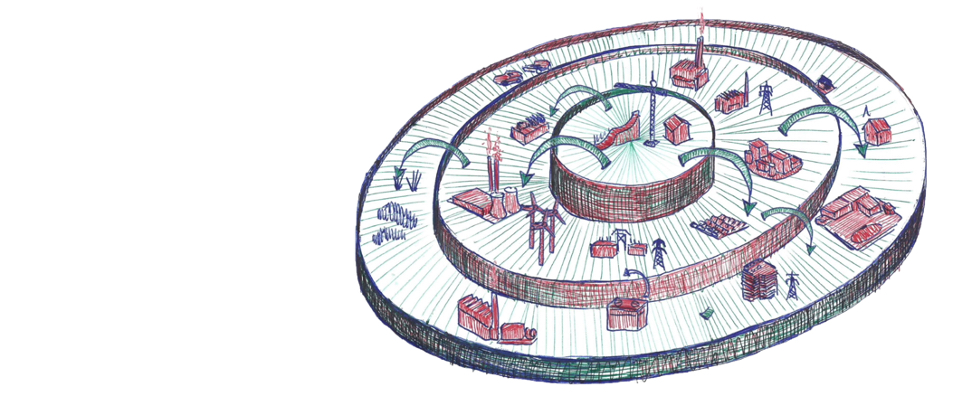
When computational models can evaluate the impact of policies objectively, why are they rarely used in public policymaking?
On 14.12.2020 by Marius SchwarzMarius Schwarz joined the Energy Science Center (ESC) in March 2020, as the project manager for Nexus-e, an energy systems modeling platform. Since 2016, Marius has been a doctoral student at Prof. Volker Hoffmann’s Group for Sustainability and Technology (SusTec). In his dissertation, he focuses on the interplay between technology diffusion and overarching systems, investigating how policies can address integration challenges of high shares of renewables and energy storage.
What should the future Swiss energy system look like? How can nuclear power be replaced? Will we have enough electricity on days where no sun is shining, no wind is blowing, and heating demand is high? Without clear answers, the discussion on the future Swiss energy system becomes bipartisan and emotional. Computational models can provide answers, facilitating a discussion less driven by subjective impressions but rather by profound technical analysis.
In 2017, the Swiss population voted for the Swiss Energy Strategy 2050. They voted to phase out nuclear power, increase decentralized electricity generation based on renewable energy, and improve energy efficiency. In 2019, Switzerland set an even more ambitious target: it pledged to be carbon-neutral by 2050. With the energy sector responsible for more than one-third of the country’s carbon emissions today, decarbonization is essential for Switzerland’s efforts to reduce its carbon footprint.
With these targets set in stone, a few questions arise: how can we advance the Swiss energy transition to achieve such targets? How can nuclear power be replaced? Will we have enough electricity on days where no sun is shining, no wind is blowing, and heating demand is high?
Answering any of these questions individually is challenging as the Swiss energy system is complex. In fact, Switzerland is highly integrated into the pan-European electricity grid and serves as Europe’s electricity hub: around 10% of the electricity exchanged between the 34 countries in Europe flows through Switzerland. On top, emerging trends such as the electrification of heating and transport have major ramifications for national energy systems, allowing the coupling of previously disconnected sectors. Most prominently is the uptake of electric vehicles and their immense charging loads, whose impact on the grid depends on how well it is managed, for example, via vehicle-to-grid. Consequently, a clear answer to the questions mentioned above is lacking. Often research only analyzes small parts of the system, resulting in opposing findings.And without clear answers, the future Swiss energy system becomes a bipartisan and emotional topic. For example, political campaigns make use of the lack of answers and have begun advocating against new energy policies.
Computational models can provide answers
Computational models are a promising option to tackle such a knowledge gap, helping decision- and opinion-making to be less driven by subjective impressions, but rather by profound technical analyses.
At ETH Zurich’s Energy Science Center, we developed Nexus-e, a modeling platform that allows us to develop scenarios for the future Swiss energy system and evaluate the impact of different policies.
In our scenarios that reflect the current regulatory landscape and expected advancements in technologies, we see trends for the development of a cost-effective and reliable future Swiss electricity system until 2050: it is mostly based on hydropower, solar power, and imports/exports, likely without additional investments into natural gas power plants. Solar power plays a central role in achieving renewable energy targets and replacing nuclear power, while new wind power would only be built if costs are reduced substantially. We also see that electricity trading with neighboring countries remains decisive, with increasing imports in winter and exports in summer.
One crucial advantage for Switzerland is its hydropower capacities. Hydro dams store water in reservoirs and adjust electricity generation in response to intermittent solar power. Hydro pumped-storage units pump water from a lower level reservoir to a higher elevation when the sun is shining and release the storage water through turbines to produce electricity when the sun goes down. However, as existing hydro power can only integrate variable solar power to a certain extent, and new plants are rarely cost-effective, other technologies such as battery storage and demand-side management become crucial. In our scenarios, we also see that Switzerland’s electricity grid is already well prepared for the shift to a decentralized power supply and that the timing of the nuclear phase-out has a limited impact.
Why are model-based scenarios rarely used in public policymaking?
When computational models like ours can evaluate the impact of policies, why are they rarely used in public policymaking? What are the challenges of using model-based scenarios, and how can we address the mistrust in scientific models?
First, models are only as good as their inputs. Developing model-based scenarios typically requires making assumptions on the future development of multiple parameters such as technology costs. While literature on technology cost developments is rich, and concepts such as the learning curve and economies-of-scale are widely used, forecasts of price developments have a long track record of being wrong. They particularly failed to foresee the rapid cost reductions of renewables.
Second, models are often inscrutable “black boxes,” with little to no possibility to evaluate model quality, data sources, and critical assumptions. Therefore, literature calls for openly-available models to foster quality science, enhance collaboration, increase productivity, and support societal and political debates. However, there are also valid reasons for not making models open-access, such as ethical and security concerns of sensitive data, unwanted exposure to code and data, additional workload, and institutional or personal inertia.
Third, models often lack extensive validation. In model validation, researchers compare model results with empirically measured data, ensuring that equations and assumptions provide a good representation of observed transformations in the energy system. A challenge for energy system optimization models is that empirical research shows that real-world energy transitions are unlikely to neatly follow the sole rationale of minimal system costs.
Fourth, models typically neglect the individual, human behavior and bounded rationality, and heterogeneity across and within societies, becoming especially important with the transition towards decentralized energy systems. Closer cooperation between modelers and social scientists would better represent the broad range of technical, economic, environmental, policy, and societal factors.
Fifth, models tend to oversimplify reality. To analyze different decarbonization pathways for the energy system, existing models have traditionally focused on specific energy sectors, adopted specific research perspectives, assessed only certain technologies, or studied isolated components and factors of the energy system. However, few efforts have been undertaken to model a broader picture of the energy-economic system successfully.
Are modeling platforms a way to go forward?
With Nexus-e, we do a first step to tackle these challenges. We build a modeling platform based on the principles of transparency, modularity, and predefined interfaces. Using the platform, we combine multiple models to represent the full spectrum of the energy system. It also allows us to bring together researchers with varying backgrounds, such as engineering and economics. We envision this platform to serve as a generally accepted, hands-on test environment for industry partners, researchers, and students. Collaborating with us on the Nexus-e platform, you can use it to answer your questions relevant to Switzerland’s and Europe’s energy future.
Therefore, I call for more models operating on Nexus-e to address the drawbacks mentioned above and bring model-based scenarios into societal discussions and public policymaking. While developing and maintaining multiple models and working in an interdisciplinary team requires extensive coordination, an existing modeling platform allows individual researchers to investigate emerging phenomena more efficiently. There should be multiple competing models in a modeling platform rather than a single one, to challenge each other’s assumptions, codes, and results and to stimulate continuous model developments that could ultimately help Switzerland achieve its 2050 goals.
Keep up with the Energy Blog @ ETH Zurich on Twitter @eth_energy_blog.
Suggested citation: Schwarz, Marius. “When computational models can evaluate the impact of policies objectively, why are they rarely used in public policymaking?”, Energy Blog @ ETH Zurich, ETH Zurich, December 14, 2020, https://blogt.ethz.ch/energy/computational-energy-models/
If you are part of ETH Zurich, we invite you to contribute with your findings and your opinions to make this space a dynamic and relevant outlet for energy insights and debates. Find out how you can contribute and contact the editorial team here to pitch an article idea!